原文链接:
端到端光学算法联合设计(end-to-end camera design)是近年来新兴起的热点分支,对一个成像系统而言,通过突破光学设计和图像后处理之间的壁垒,找到光学和算法部分在硬件成本、加工可行性、体积重量、成像质量、算法复杂度以及特殊功能间的最佳折中,从而实现在设计要求下的最优方案。端到端光学算法联合设计的突破为手机厂商、工业、车载、空天探测、国防等领域提供了简单化的全新解决方案,在降低光学设计对人员经验依赖的同时,将图像后处理同时自动优化,为相机的设计提供了更多的自由度,也将轻量化、特殊功能等计算摄影问题提供了全新的解决思路。其技术路线如图2所示。
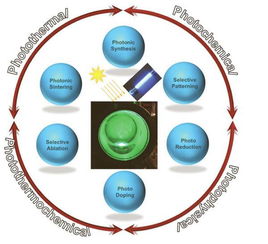
高动态范围成像(high dynamic range imaging,HDR)在计算图形学与摄影中,是用来实现比普通数位图像技术更大曝光动态范围(最亮和最暗细节的比率)的技术。摄影中,通常用曝光值(Exposure Value,EV)的差来描述动态范围,1EV对应于两倍的曝光比例并通常被称为一档(1 stops)。自然场景最大动态范围约22档,城市夜景可达约40档,人眼可以捕捉约10~14档的动态范围。
高动态范围成像一般指动态范围大于13档或8000:1(78dB),主要包括获取、处理、存储、显示等环节。
高动态范围成像旨在获取更亮和更暗处细节,从而带来更丰富的信息,更震撼的视觉冲击力。高动态范围成像不仅是目前手机相机核心竞争力之一,也是工业、车载相机的基本要求。其技术路线如图3所示。
光场成像(light field imaging,LFI)能够同时记录光线的空间位置和角度信息,是三维测量的一种新方法。经过近些年的发展,逐渐成为一种新兴的非接触式测量技术,自从摄影被发明以来,图像捕捉就涉及在场景的二维投影中获取信息。
然而,光场不仅提供二维投影,还增加了另一个维度,即到达该投影的光线的角度。
光场拥有关于光阵列方向和场景二维投影的信息,并且可以实现不同的功能。例如,可以将投影移动到不同的焦距,这使用户能够在采集后自由地重新聚焦图像。此外,还可以更改捕获场景的视角。
目前已逐渐应用于工业、虚拟现实、生命科学和三维流动测试等领域,帮助快速获得真实的光场信息和复杂三维空间信息。其技术路线如图4所示。图中所列参考文献·光场算法[1]Levoy M, Zhang Z, McDowall I.Recording and controlling the 4D light field in a microscope using microlens arrays[J].//Journal of microscopy, 2009, 235: 144-162.[2]Cheng Z, Xiong Z, Chen C, et al. Light Field Super-Resolution: A Benchmark[C] //Proceedings of the IEEE Conference on Computer Vision and Pattern Recognition Workshops. 2019.[3]Lim J G, Ok H W, Park B K, et al. Improving the spatail resolution based on 4D light field data[C]//2009 16th IEEE International Conference on Image Processing . IEEE, 2009: 1173-1176.[4]Georgiev T, Chunev G, Lumsdaine A.Superresolution with the focused plenoptic camera[C] //Computational Imaging IX.International Society for Optics and Photonics, 2011, 7873: 78730X.[5]Alain M, Smolic A.Light field super-resolution via LFBM5D sparse coding[C]//2018 25th IEEE international conference on image processing .IEEE, 2018: 2501-2505.[6]Rossi M, Frossard P.Graph-based light field super-resolution[C]//2017 IEEE 19th International Workshop on Multimedia Signal Processing .IEEE, 2017: 1-6.[7]Yoon Y, Jeon H G, Yoo D, et al. Learning a deep convolutional network for light-field image super-resolution[C]//Proceedings of the IEEE international conference on computer vision workshops. 2015: 24-32.[8]Goldluecke B.Globally consistent depth labeling of 4D light fields[C]// Computer Vision and Pattern Recognition.IEEE, 2012:41-48.[9]Wanner S, Goldluecke B.Variational Light Field Analysis for Disparity Estimation and Super-Resolution[J].//IEEE Transactions on Pattern Analysis & Machine Intelligence, 2014, 36:606-619.[10]Tao M W, Hadap S, Malik J, et al. Depth from Combining Defocus and Correspondence Using Light-Field Cameras[C] // IEEE International Conference on Computer Vision. IEEE, 2013:673-680.[11]Jeon H G, Park J, Choe G, et al. Accurate depth map estimation from a lenslet light field camera[C] // Computer Vision and Pattern Recognition. IEEE, 2015:1547-1555.[12]Neri A, Carli M, Battisti F.A multi-resolution approach to depth field estimation in dense image arrays[C] //IEEE International Conference on Image Processing.IEEE, 2015:3358-3362.[13]Strecke M, Alperovich A, Goldluecke B. Accurate Depth and Normal Maps from Occlusion-Aware Focal Stack Symmetry[C] //Computer Vision and Pattern Recognition. IEEE, 2017:2529-2537.[14]Dansereau D G, Pizarro O, Williams S B. Decoding, calibration and rectification for lenselet-based plenoptic cameras[C] //Proceedings of the IEEE conference on computer vision and pattern recognition. 2013: 1027-1034.[15]Nousias S, Chadebecq F, Pichat J, et al. Corner-based geometric calibration of multi-focus plenoptic cameras[C] //Proceedings of the IEEE International Conference on Computer Vision. 2017: 957-965.[16]Zhu H, Wang Q.Accurate disparity estimation in light field using ground control points[J].//Computational Visual Media, 2016, 2:1-9.[17]Zhang, S., Sheng, H., Li, C., Zhang, J.and Xiong, Z., 2016.Robust depth estimation for light field via spinning parallelogram operator.//Computer Vision and Image Understanding, 145, pp.148-159.[18]Zhang Y, Lv H, Liu Y, Wang H, Wang X, Huang Q, Xiang X, Dai Q.Light-field depth estimation via epipolar plane image analysis and locally linear embedding.IEEE Transactions on Circuits and Systems for Video Technology[J].2016, 27:739-47.[19]Ma H , Qian Z , Mu T , et al.Fast and Accurate 3D Measurement based on Light-Field Camera and Deep Learning[J].//Sensors, 2019, 19:4399.·光场应用[1]Lin X, Wu J, Zheng G, Dai Q. 2015. Camera array based light field microscopy. Biomedical Optics Express, 6: 3179-89[2]Shi, S., Ding, J., New, T.H.and Soria, J., 2017.Light-field camera-based 3D volumetric particle image velocimetry with dense ray tracing reconstruction technique.//Experiments in Fluids, 58, pp.1-16.[3]Shi, S., Wang, J., Ding, J., Zhao, Z.and New, T.H., 2016.Parametric study on light field volumetric particle image velocimetry.Flow Measurement and Instrumentation, 49, pp.70-88.[4]Shi, S., Ding, J., Atkinson, C., Soria, J.and New, T.H., 2018.A detailed comparison of single-camera light-field PIV and tomographic PIV.Experiments in Fluids, 59, pp.1-13.[5]Shi, S., Ding, J., New, T.H., Liu, Y.and Zhang, H., 2019.Volumetric calibration enhancements for single-camera light-field PIV.Experiments in Fluids, 60, p.21.光谱成像(spectrum imaging)由传统彩色成像技术发展而来,能够获取目标物体的光谱信息。每个物体都有自己独特的光谱特征,就像每个人拥有不同的指纹一样,光谱也因此被视为目标识别的“指纹”信息。
通过获取目标物体在连续窄波段内的光谱图像,组成空间维度和光谱维度的数据立方体信息,可以极大地增强目标识别和分析能力。光谱成像可作为科学研究、工程应用的强有力工具,已经广泛应用于军事、工业、民用等诸多领域,对促进社会经济发展和保障国家安全具有重要作用。例如,光谱成像对河流、沙土、植被、岩矿等地物都具有很好的识别效果,因此在精准农业、环境监控、资源勘查、食品安全等诸多方面都具有重要应用。特别地,光谱成像还有望用于手机、自动驾驶汽车等终端。
当前,光谱成像已成为计算机视觉和图形学研究的热点方向之一。无透镜成像(lensless imaging)技术为进一步压缩成像系统的尺寸提供了一种全新的思路(Boominathan等,2022)。传统的成像系统依赖点对点的成像模式,其系统极限尺寸仍受限于透镜的焦距、孔径、视场等核心指标。无透镜成像摒弃了传统透镜中点对点的映射模式,而是将物空间的点投影为像空间的特定图案,不同物点在像面叠加编码,形成了一种人眼无法识别,但计算算法可以通过解码复原图像信息。
其在紧凑性方面具有极强的竞争力,而且随着解码算法的发展,其成像分辨率也得到大大提升。因此,在可穿戴相机、便携式显微镜、内窥镜、物联网等应用领域极具发展潜力。另外,其独特的光学加密功能,能够对目标中敏感的生物识别特征进行有效保护,在隐私保护的人工智能成像方面也具有重要意义。
低光照成像(low light imaging)也是计算摄影里的研究热点一。手机摄影已经成为了人们用来记录生活的最常用的方式之一,手机的摄像功能也是每次发布会的看点,夜景模式也成了各大手机厂商争夺的技术制高点。不同手机的相机在白天的强光环境下拍照差异并不明显,然而在夜晚弱光情况下则差距明显。
其原因是,成像依赖于镜头收集物体发出的光子,且传感器由光电转换、增益、模数转换一系列过程会有不可避免的噪声;白天光线充足,信号的信噪比高,成像质量很高;晚上光线微弱,信号的信噪比下降数个数量级,成像质量低;部分手机搭载使用计算摄影算法的夜景模式,比如基于单帧、多帧、RYYB阵列等的去噪,有效地提高了照片的质量。但目前依旧有很大的提升空间。低光照成像按照输入分类可以分为单帧输入、多帧输入、 闪光灯辅助拍摄和传感器技术,技术路线如图2所示。技术路线如图5所示。
图中所列参考文献(向上滑动即可查看全部)[1]Padilla, D.D. and Davidson, P., 2005. Advancements in sensing and perception using structured lighting techniques: An ldrd final report.[2]Wang, J., Sankaranarayanan, A.C., Gupta, M. and Narasimhan, S.G., 2016, October. Dual structured light 3d using a 1d sensor. In European Conference on Computer Vision . Springer[3]Matsuda, N., Cossairt, O. and Gupta, M., 2015, April. Mc3d: Motion contrast 3d scanning. In 2015 IEEE International Conference on Computational Photography . IEEE.[4]O'Toole, M., Achar, S., Narasimhan, S.G. and Kutulakos, K.N., 2015. Homogeneous codes for energy-efficient illumination and imaging. ACM Transactions on Graphics , 34, pp.1-13.[5]Supreeth Achar, Joseph R. Bartels, William L. ‘Red’ Whittaker, Kiriakos N. Kutulakos, Srinivasa G. Narasimhan. 2017, "Epipolar Time-of-Flight Imaging", ACM SIGGRAPH [6]Gupta, M., Yin, Q. and Nayar, S.K., 2013. Structured light in sunlight. In Proceedings of the IEEE International Conference on Computer Vision .[7]Wang, J., Bartels, J., Whittaker, W., Sankaranarayanan, A.C. and Narasimhan, S.G., 2018. Programmable triangulation light curtains. In Proceedings of the European Conference on Computer Vision .[8]Bartels, J.R., Wang, J., Whittaker, W. and Narasimhan, S.G., 2019. Agile depth sensing using triangulation light curtains. In Proceedings of the IEEE/CVF International Conference on Computer Vision .[9]Gupta, A., Ingle, A., Velten, A. and Gupta, M., 2019. Photon-flooded single-photon 3D cameras. In Proceedings of the IEEE/CVF Conference on Computer Vision and Pattern Recognition .[10]Gupta, A., Ingle, A. and Gupta, M., 2019. Asynchronous single-photon 3D imaging. In Proceedings of the IEEE/CVF International Conference on Computer Vision .[11]Po, R., Pediredla, A. and Gkioulekas, I., 2022. Adaptive Gating for Single-Photon 3D Imaging. In Proceedings of the IEEE/CVF Conference on Computer Vision and Pattern Recognition .[12]Sun, Z., Zhang, Y., Wu, Y., Huo, D., Qian, Y. and Wang, J., 2022. Structured Light with Redundancy Codes. arXiv preprint arXiv:2206.09243.图中所列参考文献[1]Nayar, S.K., Krishnan, G., Grossberg, M.D. and Raskar, R., 2006. Fast separation of direct and global components of a scene using high frequency illumination. In ACM SIGGRAPH 2006 Papers .[2]Gu, J., Kobayashi, T., Gupta, M. and Nayar, S.K., 2011, November. Multiplexed illumination for scene recovery in the presence of global illumination. In 2011 International Conference on Computer Vision . IEEE.[3]Xu, Y. and Aliaga, D.G., 2007, May. Robust pixel classification for 3d modeling with structured light. In Proceedings of Graphics Interface 2007 .[4]Xu, Y. and Aliaga, D.G., 2009. An adaptive correspondence algorithm for modeling scenes with strong interreflections. IEEE Transactions on Visualization and Computer Graphics, 15, pp.465-480.[5]Gupta, M., Agrawal, A., Veeraraghavan, A. and Narasimhan, S.G., 2011, June. Structured light 3D scanning in the presence of global illumination. In CVPR 2011 . IEEE.[6]Sun, Z., Zhang, Y., Wu, Y., Huo, D., Qian, Y. and Wang, J., 2022. Structured Light with Redundancy Codes. arXiv preprint arXiv:2206.09243.[7]Chen, T., Seidel, H.P. and Lensch, H.P., 2008, June. Modulated phase-shifting for 3D scanning. In 2008 IEEE Conference on Computer Vision and Pattern Recognition . IEEE.[8]Couture, V., Martin, N. and Roy, S., 2011, November. Unstructured light scanning to overcome interreflections. In 2011 International Conference on Computer Vision . IEEE.[9]Gupta, M. and Nayar, S.K., 2012, June. Micro phase shifting. In 2012 IEEE Conference on Computer Vision and Pattern Recognition . IEEE.[10]Moreno, D., Son, K. and Taubin, G., 2015. Embedded phase shifting: Robust phase shifting with embedded signals. In Proceedings of the IEEE Conference on Computer Vision and Pattern Recognition .[11]O'Toole, M., Mather, J. and Kutulakos, K.N., 2014. 3d shape and indirect appearance by structured light transport. In Proceedings of the IEEE Conference on Computer Vision and Pattern Recognition .[12]O'Toole, M., Achar, S., Narasimhan, S.G. and Kutulakos, K.N., 2015. Homogeneous codes for energy-efficient illumination and imaging. ACM Transactions on Graphics , 34, pp.1-13.[13]Wang, J., Bartels, J., Whittaker, W., Sankaranarayanan, A.C. and Narasimhan, S.G., 2018. Programmable triangulation light curtains. In Proceedings of the European Conference on Computer Vision .[14]Naik, N., Kadambi, A., Rhemann, C., Izadi, S., Raskar, R. and Bing Kang, S., 2015. A light transport model for mitigating multipath interference in time-of-flight sensors. In Proceedings of the IEEE Conference on Computer Vision and Pattern Recognition .[15]Gupta, M., Nayar, S.K., Hullin, M.B. and Martin, J., 2015. Phasor imaging: A generalization of correlation-based time-of-flight imaging. ACM Transactions on Graphics , 34, pp.1-18.[16]Narasimhan, S.G., Nayar, S.K., Sun, B. and Koppal, S.J., 2005, October. Structured light in scattering media. In Tenth IEEE International Conference on Computer Vision Volume 1 . IEEE.[17]Satat, G., Tancik, M. and Raskar, R., 2018, May. Towards photography through realistic fog. In 2018 IEEE International Conference on Computational Photography . IEEE.[18]Wang, J., Sankaranarayanan, A.C., Gupta, M. and Narasimhan, S.G., 2016, October. Dual structured light 3d using a 1d sensor. In European Conference on Computer Vision . Springer.[19]Erdozain, J., Ichimaru, K., Maeda, T., Kawasaki, H., Raskar, R. and Kadambi, A., 2020, October. 3d Imaging For Thermal Cameras Using Structured Light. In 2020 IEEE International Conference on Image Processing . IEEE.计算摄影学(computational photography)是计算成像的一个分支学科,它从传统摄影学发展而来。传统摄影学主要着眼于使用光学器件更好地进行成像,如佳能、索尼等相机厂商对于镜头的研究;与之相比,计算摄影学则更侧重于使用数字计算的方式进行图像拍摄。在过去10年中,随着移动端设备计算能力的迅速发展,手机摄影逐渐成为了计算摄影学研究的主要方向:在光学镜片的物理尺寸、成像质量受限的情况下,如何使用合理的计算资源,绘制出用户最满意的图像。
计算摄影学在近年来得到了长足的发展,其研究问题的范围也所有扩展,如:夜空摄影、人脸重光照、照片自动美化等。受图像的算法,其中重点介绍:自动白平衡、自动对焦、人工景深模拟以及连拍摄影。篇幅所限,本报告中仅介绍目标为还原拍摄真实场景的真实信息的相关研究。